7 Reasons Most ML Funds Fail
In 2018, we attended Quantcon (hosted by the then famous Quantopian). The closing lecture was titled the 7 reasons most machine learning funds fail. It was at this moment that we (H&T) was introduced to the body of research by Prof. Marcos Lopez de Prado.
At the time, we were working at a pioneering hedge fund using machine learning and were very familiar with the problems we faced, what made this lecture special is that Prof. Lopez de Prado introduced solutions to these problems!
Note
Underlying Literature
Based on a popular paper, published in the Journal of Portfolio Management: The 10 Reasons Most Machine Learning Funds Fail.
We highly recommend you read the original paper as it provides a lot more depth, below are the 7 reasons:
Working in Silos (The Sisyphean Quants)
Integer Differentiation (Stationarity vs. Memory Dilemma)
Inefficient Sampling (Financial Data Structures)
Wrong Labeling (Triple-Barrier and Meta-Labeling)
Weighting of non-IID samples (Sequential Bootstrap)
Cross-Validation Leakage (Purged and Embargo CV)
Backtest Overfitting
Presentation Slides
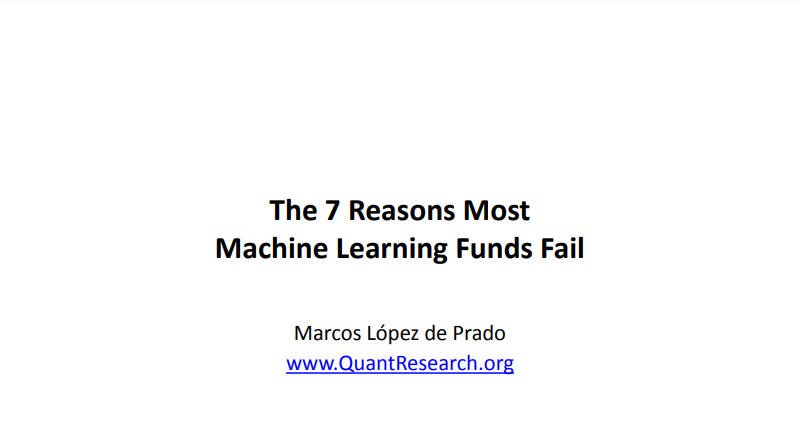
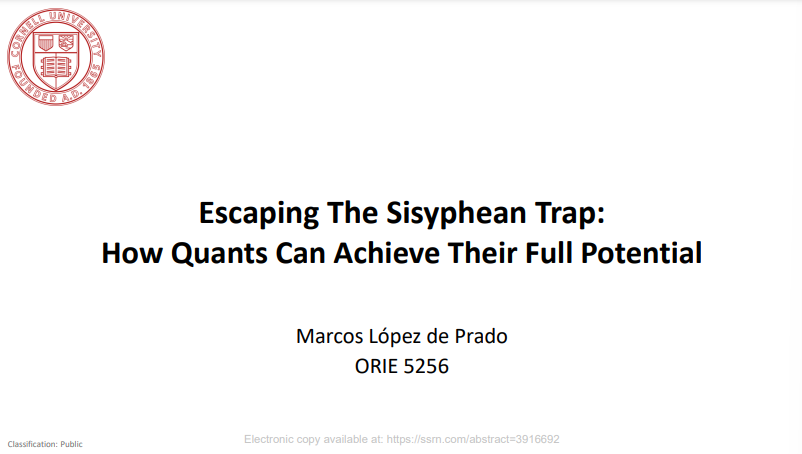